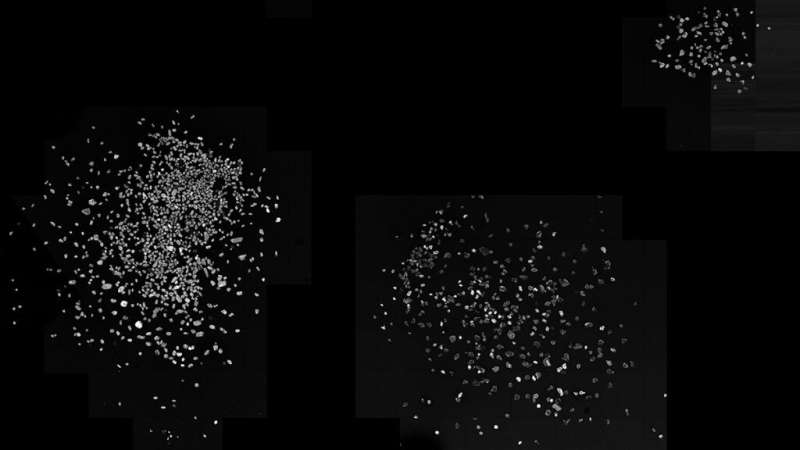
Zircons are common, hardy minerals that can be found in rocks up to 4 billion years old. Their structure and texture can reflect the conditions in which they formed, earning them a reputation as nature's time capsules. And according to new research, with the power of machine learning, scientists can mine zircon textures to identify valuable mineral deposits.
In a new study published in the Journal of Geophysical Research: Solid Earth, Chetan Nathwani and colleagues developed a method to distinguish minute differences between zircon grains formed in copper-associated rocks and granitic rocks. Their method could help scientists search for mineral deposits and probe the origins of different sediments.
The researchers used a machine learning tool called a convolutional neural network (CNN), which specializes in image analysis. Using samples collected in southern Peru, a region that produces much of the world's copper, they found that the CNN could identify shapes and textures unique to zircons found near copper deposits. The model could also distinguish these copper-associated zircons from zircons found in other kinds of rock in the region with an 85% success rate.
Copper has broad industrial applications, from electronics to construction, and the study suggests that pairing machine learning with more traditional techniques could make it easier to explore and identify copper deposits.
More information: Chetan L. Nathwani et al, Mineral Texture Classification Using Deep Convolutional Neural Networks: An Application to Zircons From Porphyry Copper Deposits, Journal of Geophysical Research: Solid Earth (2023). DOI: 10.1029/2022JB025933
Provided by Eos
This story is republished courtesy of Eos, hosted by the American Geophysical Union. Read the original story here.
Citation: Prospecting for copper with machine learning and zircons (2023, February 24) retrieved 24 February 2023 from https://phys.org/news/2023-02-prospecting-copper-machine-zircons.html
This document is subject to copyright. Apart from any fair dealing for the purpose of private study or research, no part may be reproduced without the written permission. The content is provided for information purposes only.